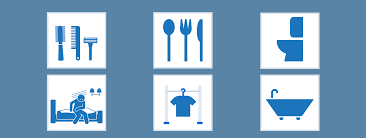
AI-Powered ADL Tracking: The Future of MDS Accuracy in Nursing Homes
Artificial Intelligence (AI) is reshaping healthcare, and long-term care is no exception. While Large Language Models (LLMs) have captured recent attention, AI-driven interventions have been making quiet but steady progress in nursing homes for years. A key area of innovation is the use of AI-enabled cameras and wearables to track Activities of Daily Living (ADLs)—a critical component of both care planning and Minimum Data Set (MDS) assessments.
The Challenge: ADL Documentation & MDS Accuracy
Accurately assessing a resident’s functional status is essential for care planning, reimbursement, and compliance. The MDS relies on observational data to determine a resident’s ability to perform ADLs like bathing, dressing, toileting, transferring, mobility, and eating. However, traditional ADL tracking depends on staff documentation, which is often subjective, inconsistent, and prone to inaccuracies due to time constraints and recall bias.
If AI-enhanced tools could provide continuous, objective ADL monitoring, they could significantly improve the accuracy of MDS assessments, reduce documentation burdens, and ensure more personalized, data-driven care.
AI-Enabled Cameras vs. Wearables: Which Is Better?
Two primary technologies are emerging in the AI-driven ADL tracking space:
- AI-Enabled Cameras (Without Directly Capturing Residents’ Faces or Identities)
- Cameras placed in resident rooms or common areas can analyze movement patterns, posture changes, and activity levels using AI.
- These systems can detect when a resident struggles with transfers, stops eating mid-meal, or requires assistance with toileting—all key factors in ADL scoring.
- Privacy concerns are mitigated by using pose detection and silhouette-based tracking rather than full video recordings.
- Wearable Sensors (Smartwatches, Clothing, or Tags)
- Residents wear discreet motion sensors that detect mobility, balance, toileting frequency, and physical activity levels.
- These devices provide real-time data on gait speed, movement assistance needs, and even meal participation through wrist-based tracking.
- Unlike cameras, wearables give individualized data and work even when residents move throughout the facility.
Each approach has its strengths—cameras provide facility-wide monitoring without resident compliance, while wearables offer granular, resident-specific data.
Implications for MDS and Reimbursement
- Improved Accuracy in ADL Scoring
- AI eliminates guesswork by automatically tracking whether residents are independent, require supervision, or need full assistance.
- Real-time data ensures that overnight challenges (like nighttime wandering or bathroom difficulties)—often missed by staff—are included in assessments.
- Reduced Staff Documentation Burden
- AI tracking auto-populates ADL scores, reducing staff workload and potential errors from memory-based reporting.
- Enhanced Risk Detection & Early Interventions
- If a resident begins toileting less frequently, AI can flag potential dehydration, UTIs, or mobility decline.
- A sudden change in gait or bed-exit frequency could indicate an increased fall risk, allowing for preventive action.
- Compliance & Regulatory Considerations
- CMS and state surveyors will need to adapt policies to account for AI-driven ADL tracking.
- Facilities may need to determine how AI-generated data integrates with the current MDS process—will it supplement or fully replace human observation?
The Future of AI in Long-Term Care
With increasing staffing shortages and regulatory scrutiny, AI-powered ADL tracking could become a vital tool for enhancing resident care, improving reimbursement accuracy, and streamlining operations in skilled nursing facilities. However, successful implementation will require balancing technology, privacy, and regulatory adaptation.
As AI becomes more embedded in long-term care, how will regulators and providers adapt? What new best practices will emerge for integrating AI-driven ADL tracking into care plans and MDS documentation?
The conversation is just beginning.